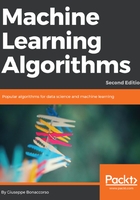
Data scaling and normalization
A generic dataset (we assume here that it is always numerical) is made up of different values that can be drawn from different distributions, having different scales and, sometimes, there are also outliers. A machine learning algorithm isn't naturally able to distinguish among these various situations, and therefore, it's always preferable to standardize datasets before processing them. A very common problem derives from having a non zero mean and a variance greater than 1. In the following graph, there's a comparison between a raw dataset and the same dataset scaled and centered:

This result can be achieved by using the StandardScaler class (which implements feature-wise scaling):
from sklearn.preprocessing import StandardScaler
ss = StandardScaler()
scaled_data = ss.fit_transform(data)
It's possible to specify if the scaling process must include both mean and standard deviation by using the with_mean=True/False and with_std=True/False parameters (by default, they're both active). If you need a more powerful scaling feature, with a superior control on outliers and the possibility to select a quantile range, there's also the RobustScaler class. Here are some examples with different quantiles:
from sklearn.preprocessing import RobustScaler
rb1 = RobustScaler(quantile_range=(15, 85))
scaled_data1 = rb1.fit_transform(data)
rb1 = RobustScaler(quantile_range=(25, 75))
scaled_data1 = rb1.fit_transform(data)
rb2 = RobustScaler(quantile_range=(30, 60))
scaled_data2 = rb2.fit_transform(data)
The results are shown in the following graphs:

Other options include MinMaxScaler and MaxAbsScaler, which scale data by removing elements that don't belong to a given range (the former) or by considering a maximum absolute value (the latter).
The scikit-learn library also provides a class for per-sample normalization, Normalizer. It can apply Max, L1, and L2 norms to each element of a dataset. In a Euclidean space, these transformations are defined in the following way:
An example of every normalization is shown in the following snippet:
from sklearn.preprocessing import Normalizer
data = np.array([1.0, 2.0])
n_max = Normalizer(norm='max')
n_max.fit_transform(data.reshape(1, -1))
[[ 0.5, 1. ]]
n_l1 = Normalizer(norm='l1')
n_l1.fit_transform(data.reshape(1, -1))
[[ 0.33333333, 0.66666667]]
n_l2 = Normalizer(norm='l2')
n_l2.fit_transform(data.reshape(1, -1))
[[ 0.4472136 , 0.89442719]]